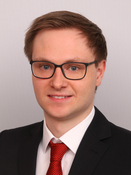
M. Sc. Philipp Karg
- Research Associate
- Group:
- Room: 206
- Phone: +49 721 608-42708
- Fax: +49 721 608-42707
- philipp karg ∂does-not-exist.kit edu
Karlsruher Institut für Technologie (KIT) Campus Süd
Institut für Regelungs- und Steuerungssysteme
Geb. 11.20 (Engler-Villa)
Kaiserstr. 12
D-76131 Karlsruhe
Curriculum Vitae
Studies of electrical engineering and information technology at the Karlsruhe Institute of Technology (KIT). Bachelor’s thesis at the Institute of Control Systems (IRS) on load forecasting in electricity distribution grids with high renewable energy penetration (2016). Following this, master’s studies with focus on control engineering and systems theory. Internship at the Autonomous Driving Campus of BMW in Unterschleißheim in the field of simulative validation of highly automated driving functions. Master’s thesis at the IRS on exploration strategies for cooperative controllers based on reinforcement learning methods (2019).
Since July 2019, member of the scientific staff of the Institute of Control Systems.
Research
Modeling and Identification in Cooperative Human-Machine Scenarios
In almost all industrial sectors and even in the everyday life the amount of automated tasks increases. This leads to significant changes in the working and everyday environment. However, since the automation mainly concerns tasks and not complete jobs, humans more and more have to interact with highly automated systems. Examples can be found in the field of medical engineering, manufacturing engineering (fenceless factory) or automotive engineering.
In such situations, the human and the automation influence a technical system mutually and equally. In order to design the automation in such a cooperative human-machine scenario properly, a description resp. model of the human behavior is an important first step. Then, based on this model an identification algorithm will be needed to determine the crucial parameters, which describe how the human behaves. Designing the automation based on such an identified model provides the opportunity to get the most of the synergies of the cooperation.
Within the research project, describing the human as an optimal controller acts as a promising modeling approach. Thereby, the variability of human movements leads to additional challenges. In context of the research project, this variability is to be integrated and analyzed in the identification algorithms. A promising starting point is given by methods relating to Inverse Reinforcement Learning.
Publications
Kille, S.; Leibold, P.; Karg, P.; Varga, B.; Hohmann, S.
2024. 33rd IEEE International Conference on Robot and Human Interactive Communication (ROMAN 2024), 1595–1602, Institute of Electrical and Electronics Engineers (IEEE). doi:10.1109/RO-MAN60168.2024.10731297
Karg, P.; Kienzle, A.; Kaub, J.; Varga, B.; Hohmann, S.
2024. IEEE Conference on Control Technology and Applications (CCTA 2024), 824–831, Institute of Electrical and Electronics Engineers (IEEE). doi:10.1109/CCTA60707.2024.10666619
Karg, P.; Hess, M.; Varga, B.; Hohmann, S.
2024. 2024 European Control Conference (ECC), 537–544, Institute of Electrical and Electronics Engineers (IEEE). doi:10.23919/ECC64448.2024.10591104
Karg, P.; Stoll, S.; Rothfuß, S.; Hohmann, S.
2023. IFAC-PapersOnLine, 56 (2), 8320–8326. doi:10.1016/j.ifacol.2023.10.1021
Karg, P.; Stoll, S.; Rothfus, S.; Hohmann, S.
2023. 2022 IEEE 61st Conference on Decision and Control (CDC), 2801–2808, Institute of Electrical and Electronics Engineers (IEEE). doi:10.1109/CDC51059.2022.9992798
Karg, P.; Koepf, F.; Braun, C. A.; Hohmann, S.
2023. IEEE transactions on automatic control, 68 (1), 596–603. doi:10.1109/TAC.2022.3145651
Karg, P.; Stoll, S.; Rothfus, S.; Hohmann, S.
2022. doi:10.48550/arXiv.2210.17265
Karg, P.; Kalb, L.; Bengler, K.; Hohmann, S.
2021. ATZ worldwide, 123 (2), 68–72. doi:10.1007/s38311-020-0609-y
Karg, P.; Kalb, L.; Bengler, K.; Hohmann, S.
2021. Automobiltechnische Zeitschrift, 123 (2), 66–70. doi:10.1007/s35148-020-0636-4
Belgardt, S.; Doer, C.; Hohmann, S.; Karg, P.; Rothfuß, S.; Siebenrock, F.; Stork, W.; Terzidis, O.; Tittel, A.; Zwick, T.
2021. Handbuch Qualität in Studium, Lehre und Forschung, 76, 67–84
Stark, O.; Karg, P.; Hohmann, S.
2020. 2020 59th IEEE Conference on Decision and Control (CDC), Jeju, Korea (South), 14-18 Dec. 2020, 5159–5166, Institute of Electrical and Electronics Engineers (IEEE). doi:10.1109/CDC42340.2020.9304346
Flad, M.; Karg, P.; Roitberg, A.; Martin, M.; Mazewitsch, M.; Lange, C.; Kenar, E.; Ahrens, L.; Flecken, B.; Kalb, L.; Karakaya, B.; Ludwig, J.; Pruksch, A.; Stiefelhagen, R.; Hohmann, S.
2020. Smart Automotive Mobility: Reliable Technology for the Mobile Human. Ed.: Gerrit Meixner, 1–70, Springer
Flad, M.; Ludwig, J.; Roitberg, A.; Karg, P.; Stiefelhagen, R.; Hohmann, S.
2019. Technische Informationsbibliothek (TIB)
Korus, J.-D.; Karg, P.; Ramos, P. G.; Schütz, C.; Zimmermann, M.; Müller, S.
2019. IFAC-PapersOnLine, 52 (8), 1–6. doi:10.1016/j.ifacol.2019.08.026
Sauter, P. S.; Karg, P.; Kluwe, M.; Hohmann, S.
2018. 2018 IEEE PES Innovative Smart Grid Technologies Conference Europe, ISGT-Europe 2018; Sarajevo; Bosnia and Herzegovina; 21 October 2018 through 25 October 2018, Art. Nr.: 8571524, Institute of Electrical and Electronics Engineers (IEEE). doi:10.1109/ISGTEurope.2018.8571524
Sauter, P.; Karg, P.; Pfeifer, M.; Kluwe, M.; Zimmerlin, M.; Leibfried, T.; Hohmann, S.
2017. Die Energiewende : Blueprints for the New Energy Age, Proceedings of the International ETG Congress 2017, World Conference Center, Bonn, 28th - 29th November 2017, 13–18, VDE Verlag